
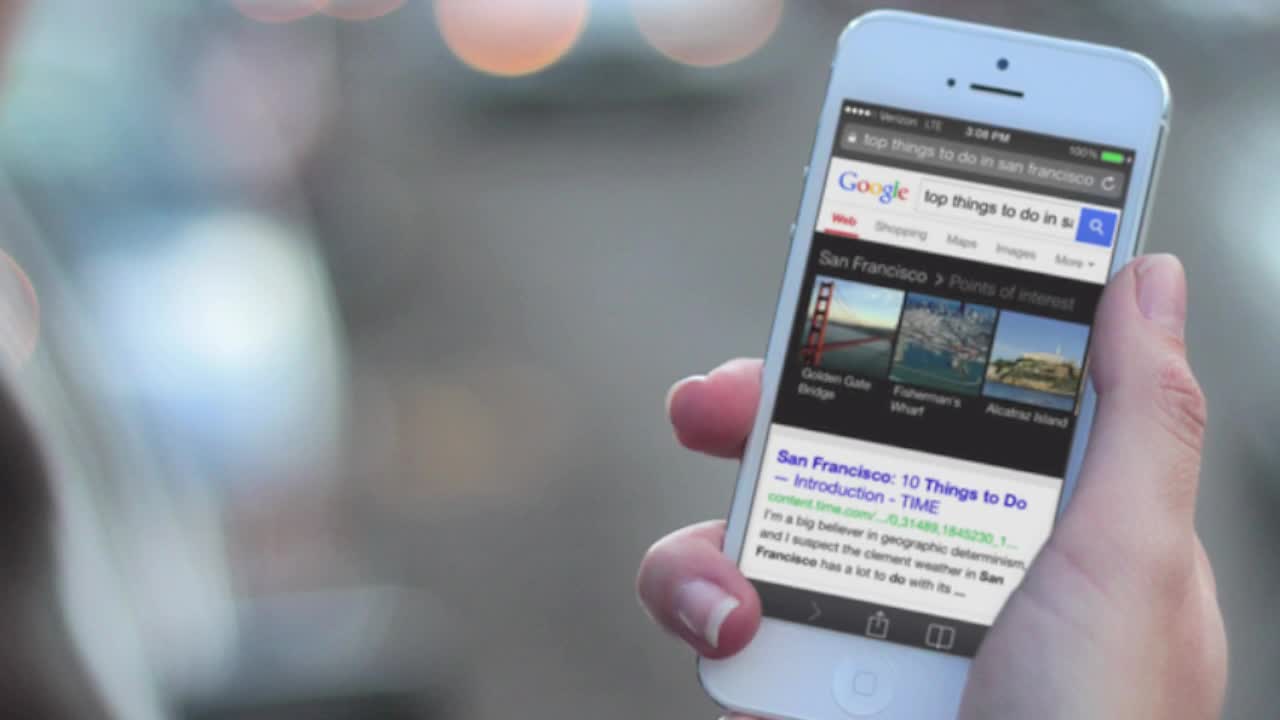
人类判断
TRANSCRIPT:
Hi, I'm Suvda Myagmar from Expect Labs and I'm continuing my talk on evaluating search quality. What is search quality? It's a measure of how relevant search results are for a given query, and how satisfied the users are with the search experience. Sometimes users' satisfaction does not necessarily correlate to high relevance of results. The user may be happy with just better presentation of the results or with more unique content instead of highly relevant popular content.
Common search quality metrics are precision and recall. Precision is percent of relevant documents in the result set or true positives. Recall is percent of all relevant documents that have been returned, basically it measures whether any relevant documents are missing. You should optimize for both precision and recall, but I believe, in web search, it's more important to optimize the precision because users rarely notice whether they're missing any relevant documents, but do notice bad documents returned. You can combine precision and recall into a single metric like accuracy and F-measure. Another common metric is NDCG (Normalized Discounted Cumulative Gain). It's computed by combining relevance ratings for each document in the result set, typically discounting for lower rank positions and normalizing across queries. NDCG has limitations where it doesn't penalize for having bad documents or for missing relevant documents in the result set.
The data necessary to compute these metrics can be collected using live A/B testing or manual rating by human judges.
Live A/B testing utilizes the wisdom of the crowd. By serving a portion of users with results from one ranking algorithm and the other with another ranking algorithm, you can collect comparative data from user logs. The user logs track a user's behavior within a search session: how long the user stayed within the session, which documents he/she clicked, which clicks bounced back etc. This method can be difficult to use for small search projects because either you don't have proper logging mechanisms to track user actions or you don't have enough data.
Next, I will talk about methods for manually rating search results.